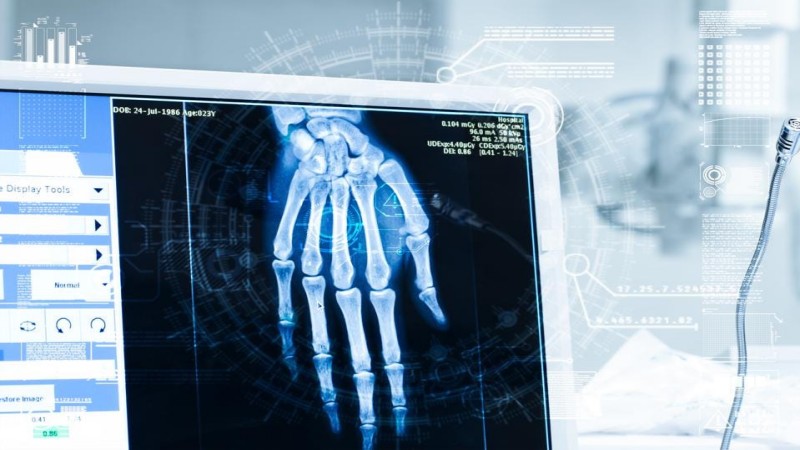
New research from WMG at the University of Warwick in the United Kingdom shows that artificial intelligence (AI) can reduce the time needed to process abnormal chest x-rays and prioritize which x-rays need to be expedited.
The research was led by Professor Giovanni Montana, Chair in Data Science in WMG at Warwick University. His team worked with Guy’s and St Thomas’ Hospitals in the UK to extract a dataset of half million anonymized adult chest X-Rays to teach the AI system to understand visual patterns in X-rays that predicted and assessed urgency levels and recognize in real time, abnormalities in those X-rays.
Montana says that by using this AI technology, a radiologist will be able to potentially detect abnormal radiological scans as soon as they are acquired, even before the patient leaves the hospital.
“This can make a tremendous difference in the diagnosis of life-threatening conditions such as lung cancer. For instance, the UK has the third lowest number of radiologists per population out of 31 European countries, and as recently as 2017 there have been cases of patients who died or suffered significant harm after their chest x-ray exams went unreported by radiologists.”
When a patient is admitted to the hospital to have a diagnostic chest x-ray, the image goes into a queue until a radiologist can visually inspect it and write a report.
Montana says that the increased demand in healthcare has put pressure on radiology service and wait times are now becoming longer and longer.
“For instance, in the UK, figures suggest the radiologist’s workload of reading and interpreting scans has increased by 30% between 2012 and 2017,” said Montana. “On the other hand, the number of consultant radiologists is increasing at a much lower rate. The longer a patient waits for a report, the higher the chances that a potentially harmful condition is diagnosed too late.”
“Our AI system provides a simple solution to this problem by offering an artificial pair of expert radiological eyes: the algorithm is executed as soon as the scan is generated at the hospital. It reads the image autonomously, without any human intervention, and can flag any critical abnormalities in nearly real-time by alerting a radiologist, e.g. by sending out a text message.”
“Delays result in missed opportunities to diagnose lung cancer and other serious conditions of the lungs and heart in a timely fashion,” said Montana. “The problem is even more severe in less developed countries worldwide where the number of radiologists per million population is substantially lower. AI systems such as this one could make a difference in the future delivery of radiological diagnosis globally.”
Montana says the teams AI algorithm learned to identify radiological abnormalities nearly as accurately as an experienced senior radiologist after several years of clinical practice. “It can reliably be used as a fully-automated triaging and prioritisation system,” added Montana.
In addition to speeding up diagnosis, Montana notes that the new AI system can also transform radiological workload.
“Since nearly 40% of all the reported chest x-rays turn out to be normal, detecting those as soon as they are acquired allows radiologists to allocate more time reviewing only the scans that require their immediate attention,” said Montana. “Because the system can pinpoint what abnormalities can be seen on the images, it will allow for a smart and efficient allocation of available expertise, for instance, a cardiovascular radiologist could be contacted directly by the system as soon as required.”
Montana says that using AI in tandem with diagnosis is part of the healthcare 4.0 trend in hospitals and laboratories. “This a representative example of how advanced data analytics and AI in digital healthcare can be deployed to positively transform the delivery of digital healthcare services and ultimately improve the quality of care provided to patients.”
“Unlike other technologies falling under the umbrella of Healthcare 4.0, such as connected wearable devices, whose adoption is currently limited by the slow pace at which the digital transformation is taking place in hospitals, these “smart radiological assistants” can be seamlessly and cheaply integrated with existing IT solutions,” added Montana.
“Currently, the main barrier to the adoption of AI systems in radiology is the lack of confidence in how these technologies work and perform, which was the main motivation behind our study,” said Montana. “I have no doubt that more radiologists will continue to build trust and become more appreciative of the advantages these systems can bring into their profession.”
The results from the paper were published in the January 2019 in the medical journal Radiology.
Jennifer Kite-Powell is a writer who covers innovation in technology and science as it intersects with industry, culture, health, environment and mobility. You can follow her on Twitter @jennalee.