Machine Learning And Artificial Intelligence In Business: Year In Review, 2018
David A. Teich – Contributor
Enterprise & Cloud
B2B technology analyst and consultant
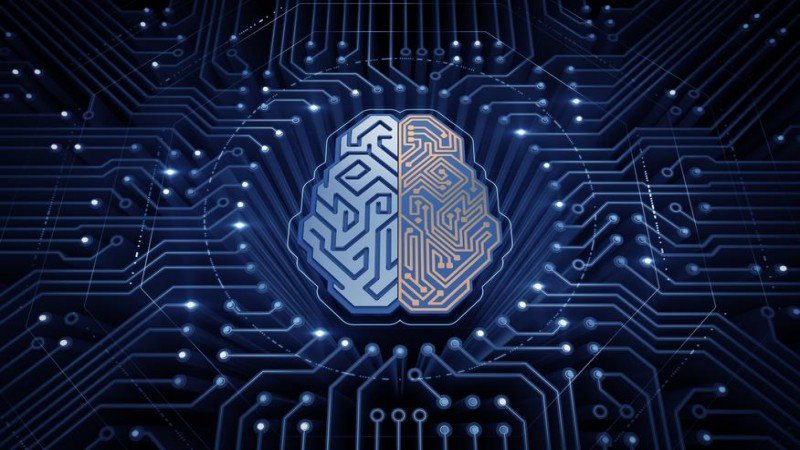
Artificial Intelligence FOTOLIA – SERGEY TARASOV
Much has happened in artificial intelligence (AI) this year. From NVIDIA NVDA +0.75%, Intel INTC +2.34% and a host of startups announcing new chips focused on both training and run time inference, to announcements of a wide variety of new algorithms, much of the news has been focused on research and academia. The challenge is to notice what is happening with AI and machine learning (ML) that business can look to for short term adoption to aid in performance.
The technologies and techniques of AI and ML are still so new that the main adopters of the techniques are the large software companies able to hire and to invest in the necessary expertise. Those companies come into focus by looking at two pairs of companies which show the two angles of attack.
There are Google GOOGL +3.21% and Amazon, early movers due to their cloud foundation who are working to figure out how to generalize techniques developed for their products, in order to attract the wider business market. On the other side are Microsoft MSFT +3.46% and IBM IBM +0.11%, companies with long histories of addressing business needs who are layering AI across product offerings. Yes, there are plenty of other companies also working to add machine learning to their product lines, and an even larger body of startups focusing on building solutions with AI and ML techniques at the core, but those four companies are well known and display the two key methods to attack the problem.
Within the new technologies, there are certain areas that have gained more traction than others that are even earlier in their lifecycles. Let us discuss a few of the areas.
Natural Language
For years, search engines have been getting better at understanding more natural syntax, both written and spoken, for questions people ask. The ability to understand more human language is natural language processing (NLP). While the two previous years saw attention on personal assistants, 2018 was the year that serious inroads into business analytic applications have been made in NLP.
Just about every business intelligence (BI) and enterprise software company released versions of NLP for their analytic applications. The ability for business line management and employees to type or speak “what’s the sales last quarter for region X?” rather than have to deal with drop down list boxes and other more technical UX features is helping move BI from shelf-ware to regular use.
On the other side, reporting is almost coming full circle. From early full text reports on mainframes, to basic graphics being added, to the current world of advanced visualizations, images have taken over in much of the analytics world. Yet people see things differently, so combining visualizations and text better serves a wider audience.
BI companies are also beginning to add natural language generation (NLG) to analytics applications. There are two key approaches to NLG. First, there is text that is built to accompany the visualizations, providing descriptions and context that helps explain those images. Second, not all analytics require visualizations, so ML analytics can process information in the background and provide language responses. Those answers can be provided in text alerts to phones, as voice response to voice queries, or in a number of other ways where visualizations aren’t necessary.
As with vision, which has seen (no pun intended) significant business expansion in the last decade, natural language is not a new part of the AI world. What has happened is that hardware has caught up to the processing power needed provide NLP and NLG in the real-time speed needed by business and consumers. This year was the first to see significant additions of natural language tools into business applications, and that will only speed up in 2019.
Machine Learning
The growth of machine learning is still primarily in the ever widening field of neural network. Unlike natural language, ML isn’t as visible to the end user, it is a core technology used to analyze information to help in understanding existing patterns and plan for future actions. Because ML is hidden from the end users, it creates a lot of opportunity for startups to try different models and techniques. Those companies can then partner with larger companies to leverage themselves into the business environment. While ML companies who market to specific niches have a chance to grow independently, the odds are that the ones who make the most inroads will be acquired by larger firms who realize that buying can be a more rapid entrance than building. Such an example was Tableau’s acquisition of Empirical Systems.
While the potential for ML is large, adoption is slow for the key reason of access to personnel. Machine learning is still very much a coding specialty, requiring frameworks such as TensorFlow, Caffe, and Spark MLlib, all of which need strong knowledge. The restricted hiring pool slow the ability for the knowledge to spread.
This problem isn’t new to ML. Business intelligence is a recent technology that saw the same growth curve. It wasn’t until companies created core tools that helped systems analysts and business users access BI technology to create their own analysis that we saw the growth of the last decade.
Until the ML industry moves past the reliance on very technical frameworks, ML adoption will primarily come from two areas:
large companies willing to pay to build a group of programmers
startups where a small group of ML people can focus on specific areas
As with other new technologies, the challenge of finding enough people to do the work means that the educational sector is getting involved. Programs such as Eastern Washington University’s Bachelor of Science in Data Analytics are beginning to push forward.
There’s nothing wrong with that, as the pattern of new technology adoption is being repeated. What it means is that ML is still early in the adoption cycle, it won’t be approaching the mass market until more companies can incorporate ML techniques into their solutions using a combination of a larger pool of trained employees and higher level tools.
Robotics
Robotics is one of the oldest branches of AI. What recent years, including 2018, have seen is that branches don’t remain independent. Advances in the other areas of AI, in networking, processing speeds, and more, have meant that robotics has seen two very interesting changes begin to occur.
First, robotics is clearly moving off of the manufacturing floor. Smaller robots and robotic processes are becoming cost effective in many areas of business and spreading their use, such as bread baking machines by Wilkinson Baking Company that fit in grocery stores.
Second, the power of networking and the cloud have combined to mean that robots can better leverage other aspects of AI in order to perform in coordination with other robots and with humans. This year’s SAP TechEd was one of a number of places showcasing early forms of robotic coordination, leveraging vision, machine learning, and other techniques to help connected robots improve performance in manufacturing, shipping and other business functions.
Robotic Process Automation
There is a new phrase/acronym that makes me uncomfortable, for a number of reasons, and it has exploded this year. Robotic Process Automation (RPA). The concept is very appealing. The idea is that software observes repeated functions in business processes, functions performed on computers. The software is intelligent, primarily leveraging an ML base. Rather than business analysts attempting to extract what people claim to be doing and then defining automated processes, the ML systems observe what people are regularly doing and then the systems can automate the tasks.
The promise is that more routine tasks can be more error prone due to human boredom, so automating those and allowing people to focus on more interesting tasks will improve performance. In addition, automating routine tasks will speed up those tasks, providing faster resolution that can better match SLA’s and improve customer relations.
The Impact Beyond 2018
The key to the RPA message is that the systems aren’t replacing people, they’re only automating the most routine tasks, allowing the skilled workers to focus on exceptions and more complex work. That’s great in theory, but it’s another step in a major economic shift that is being ignored. That leads to the question of the impact of all the changes above on the next few years. That’s complex and worth another column.
The overview, though, is simple. It is very clear that advances in technology, AI in particular, mean that many jobs will be disappearing in the next few years. Some pundits like to claim that the need to AI coders will mean jobs aren’t lost. That, however, seems to be a specious argument. When a few programmers can remove the jobs of thousands, that’s not a balance. More importantly, what is society doing in order to ensure that displaced workers can either be trained for a new economy or are taken care of in a way that a modern, civilized, society should be capable of doing.
Sure, the move from horses to cars put a lot of people out of jobs. The issue is that the move from manufacturing buggy whips to manufacturing car parts was not as big a jump as going from a manufacturing floor to working in a machine learning framework.
RPA, the other technologies mentioned above, have great potential for helping business. What’s needed is to understand that the economic changes that will follow are not something that should be ignored. When the Supreme Court can quote a comic book character, the quote is worthwhile. As Spiderman’s uncle said, “With great power comes great responsibility”. The potential of artificial intelligence and machine learning is great, 2018 saw strong advances towards that greatness, it’s not too early to think about the responsibilities.
Finally, as mentioned in the introductions, the buy-v-build decision for these new technologies is an easy decision for the big players. The were a steady dribble of early acquisitions in 2018 and the frequency of those should accelerate in 2019.
This past year saw the early stages of AI and ML adoption in the business world begin to solidify. While the growth will continue in 2019, it is still early stage growth. The thing to note about cloud applications is that not only can they ramp up more quickly than those of on-premises systems in the past, that means the early adoption portion of the curve will move more quickly. Expect to see more mainstream adoption of many techniques in the next three years.
David A. Teich is interested in business intelligence (BI), artificial intelligence (AI), machine learning (ML) and other advances technologies, focused on how they help businesses improve performance. He’s an analyst and consultant in those areas as well as in high tech.
@Teich_Comm